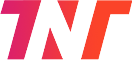
In recent years, artificial intelligence (AI) has made valuable advancements, revolutionizing different industries. However, along with its advantages, AI technology also presents challenges and risks. One such phenomenon that has garnered attention is AI hallucinations. This blog targets to explore the concept of AI hallucinations, explore how they occur, provide examples, discuss preventive measures, and highlight the role of Google Cloud in addressing this issue.
AI hallucinations refer to instances where artificial intelligence systems produce erroneous or misleading outputs, leading to incorrect interpretations or perceptions. Unlike human hallucinations, which involve sensory perceptions without external stimuli, AI hallucinations occur due to algorithmic errors or biases within AI models.
AI hallucinations can arise from various factors, including flawed data inputs, biased algorithms, or inadequate training datasets. When AI systems encounter unfamiliar or ambiguous data patterns, they may generate inaccurate predictions or classifications, resulting in hallucinatory outputs. Moreover, algorithmic biases, inadvertently encoded during the model training process, can exacerbate these hallucinations, perpetuating discriminatory or misleading results.
Also read: Top 10 Helpful GitHub Storage For Web DevelopersExamples of AI hallucinations illustrate instances where AI systems generate erroneous outputs, deviating from intended outcomes. For instance, misidentification in image recognition, nonsensical text generation in natural language processing, and misinterpretation of sensor data in autonomous vehicles exemplify common forms of AI hallucinations.
Some key strategies include.
To reduce the occasion of AI hallucinations, several strategies can be employed.
Google Cloud offers a suite of AI tools and services designed to address the challenges associated with AI hallucinations.
AI hallucinations pose significant challenges in the deployment and utilization of artificial intelligence systems. By understanding the underlying causes, exploring preventive measures, and leveraging advanced technologies like Google Cloud, and hallucinations in generative AI, organizations can mitigate the risks associated with AI hallucinations and foster the responsible and ethical development of AI technology. As AI continues to evolve, proactive efforts to address hallucinatory behaviors will be crucial in realizing its full potential while minimizing unintended consequences.
AI hallucinations refer to erroneous or misleading outputs generated by artificial intelligence systems, leading to incorrect interpretations or perceptions due to algorithmic errors or biases.
AI hallucinations can arise from factors such as flawed data inputs, biased algorithms, or inadequate training datasets, causing AI systems to produce inaccurate predictions or classifications.
Examples include misinterpretations in image recognition, sentiment analysis errors in natural language processing, and misjudgments in autonomous driving systems, all of which result in misleading or incorrect outcomes.
Prevention strategies include using diverse and representative data, implementing robust testing and validation procedures, embracing explainable AI techniques, and establishing mechanisms for continuous monitoring and feedback.
Google Cloud offers pre-trained models, AI explainability services, data integrity solutions, and tools for continuous improvement, enabling organizations to minimize the risk of AI hallucinations and enhance model reliability.
Thursday November 23, 2023
Monday November 20, 2023
Monday October 2, 2023
Wednesday September 20, 2023
Wednesday September 20, 2023
Friday September 15, 2023
Monday July 24, 2023
Friday July 14, 2023
Friday May 12, 2023
Tuesday March 7, 2023